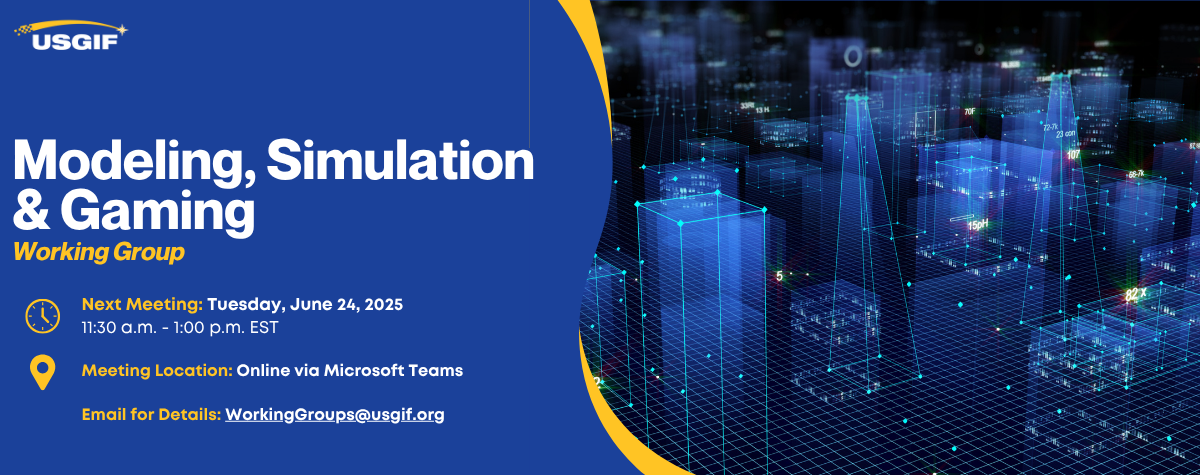
Modeling, Simulation & Gaming Working Group
The Modeling, Simulation & Gaming Working Group (MSGWG) collaborates with industry, academia, and government leaders to advance GEOINT tradecraft capable of delivering authoritative and relevant at the point of need.